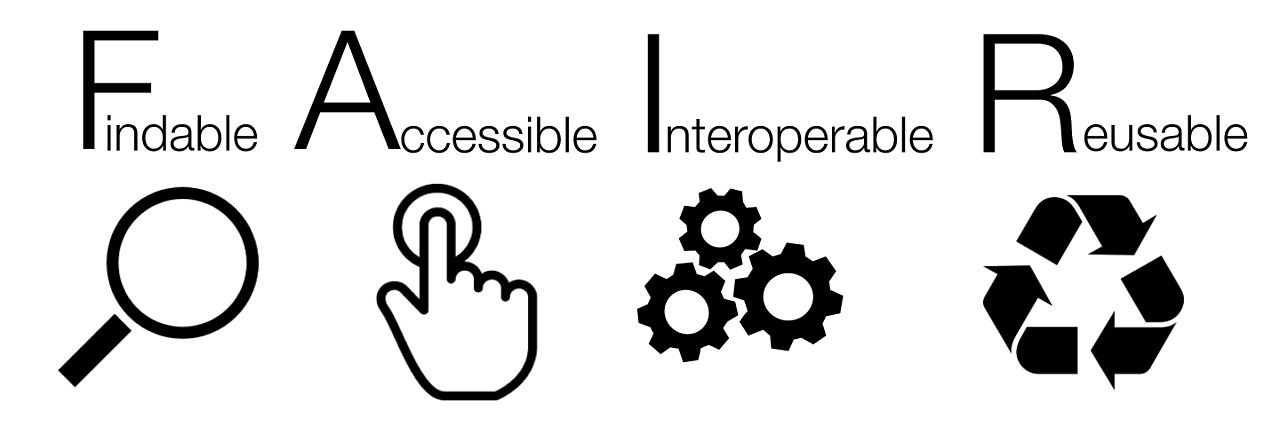
Project data management using FAIR Principles
Disciplines such as modern chemistry are data-rich; a lot of data from diverse sources is generated during the research process. During a multi-faceted project, such data whenever possible should be managed according to the so-called FAIR principles.1 This acronym means that the data should be
- Findable. The data should be searchable using well-controlled definitions of its properties, and where possible such searches should be capable of being undertaken without necessarily having access the article in which the data is discussed. The data in other words is nowadays considered a first class scientific object in its own right.
- Accessible. This means a clearly stated mechanism for retrieving the data should be available, and one moreover capable of being exploited not just be a human but by an unsupervised machine procedure which can operate on whatever scale is desired.
- Inter-operable. The properties of the data should be well enough defined that it can be subjected to operations using suitable methods and algorithms, which serve to transform it into new representations and procedures that can generate new information about the object the data is describing.
- Re-usable. The terms and conditions for interoperating the data should be clearly defined so that it can be used appropriately.
The secret to generating and managing data according to these FAIR principles is to ensure that a rich set of metadata describing the data itself is also created. Such metadata allows all four aspects outlined above. The best way of illustrating the application of FAIR principles is to show examples. Here they are illustrated in the context of amidation reactions, along with the DOI that has been obtained in exchange for registering the metadata describing the research object. Such a DOI (digital object identifier) allows access to a landing page for the data object, where information about the object can be found, along with access mechanisms for obtaining a copy.
The first principle that emerges from FAIR objects is that each type of data has its own characteristic properties and so accordingly does the metadata describing this data. It is also evident that the concept of FAIR metadata is relatively new and is currently still rapidly evolving, as are the standards that apply to it. So the exemplars here are a snapshot of this area, taken during the period 2016-2021, and which might itself be be expected to evolve and improve over time. Use these only as illustrative examples and not necessarily as standards that will be adhered to in the future.
Example 1. Research data generated from a catalytic amidation project.2
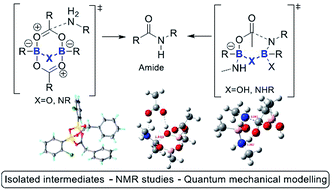
The project involved the generation of various types of chemical data, including:
- Computational data for catalytic cycles3. Conventional data for such computations as found in supporting information files is generally restricted to listing only the coordinates of the final geometry of the modelled system. The FAIR-enhanced data now additionally includes the input file used for the calculation (so that all keywords and options are available for replicability) and the outputs in two formats, a log file which summarises the progress of the calculation and a checkpoint file which includes the full final wavefunction. The latter in turn allows new properties to be derived (inter-operated) from this wavefunction by re-use of the data. Such data appears in Example 2 below.
- NMR spectra4. Such spectra are conventionally presented in supporting
information as a static PDF-based representation of the frequency domain transform of the
original free-induction-decay (FID) data obtained from the instrument. The FAIR-enhanced
version includes the complete original instrumental data (also called the raw or primary data)
and/or a packaged version produced by a specialised program available for (re)processing such
data and which can include both the FID time-domain data as well as the (Fourier) transformed
frequency domain spectrum along with any user-added annotations. Because raw NMR data is
worthless without access to such an appropriate transform program, also included in the FAIR
fileset is a free-to-use license for that specific dataset for applying the MestreNova program
to produce a spectrum.5 All you have to do to get an NMR dataset is to download the
.mnpub
file found on the landing page for the DOI, install the MestreNova program (without necessarily licensing it) and double click the.mnpub
file. The license for that dataset, as included in the.mnpub
file, allows all operations on the data that the MestreNova program is capable of. This illustrates the concept of inter-operability very nicely. - Crystal structures6. Conventional data for crystal structures is made available via the Cambridge crystal structure database in the form of final coordinates. Such data allows visualisation of the structure, but may lack the structure factors to enable a more systematic re-evaluation of the refining processes used to obtain the structure. It certainly also lacks the full image data on which the refinement was based. FAIR-enhanced versions of such data would certainly include the structure factors and may also increasingly include the raw image data from which a complete re-refinement of the structure is enabled.
- Crystal structure search queries7. If the complete Cambridge structure database has been searched for related structures as part of the report of the research, then the defined search queries can also be included as FAIR-enhanced data so that others can either repeat/re-use the search or interoperate it to define new searches based on the original.
There were other types of data reported for which FAIR methods have not yet been developed; these remain available in the conventional article supporting information.
Example 2. A research presentation exploiting FAIR data.8
A research presentation can include original data to illustrate a theme. In this example, the data for the computed transition state model for the rate determining step can be retrieved from its data repository source9 and then presented (Inter-operated and Re-used) as a 3D model in the slide.
You can also view this presentation slide directly.10
Example 3. Finding by exploiting the FAIR metadata.
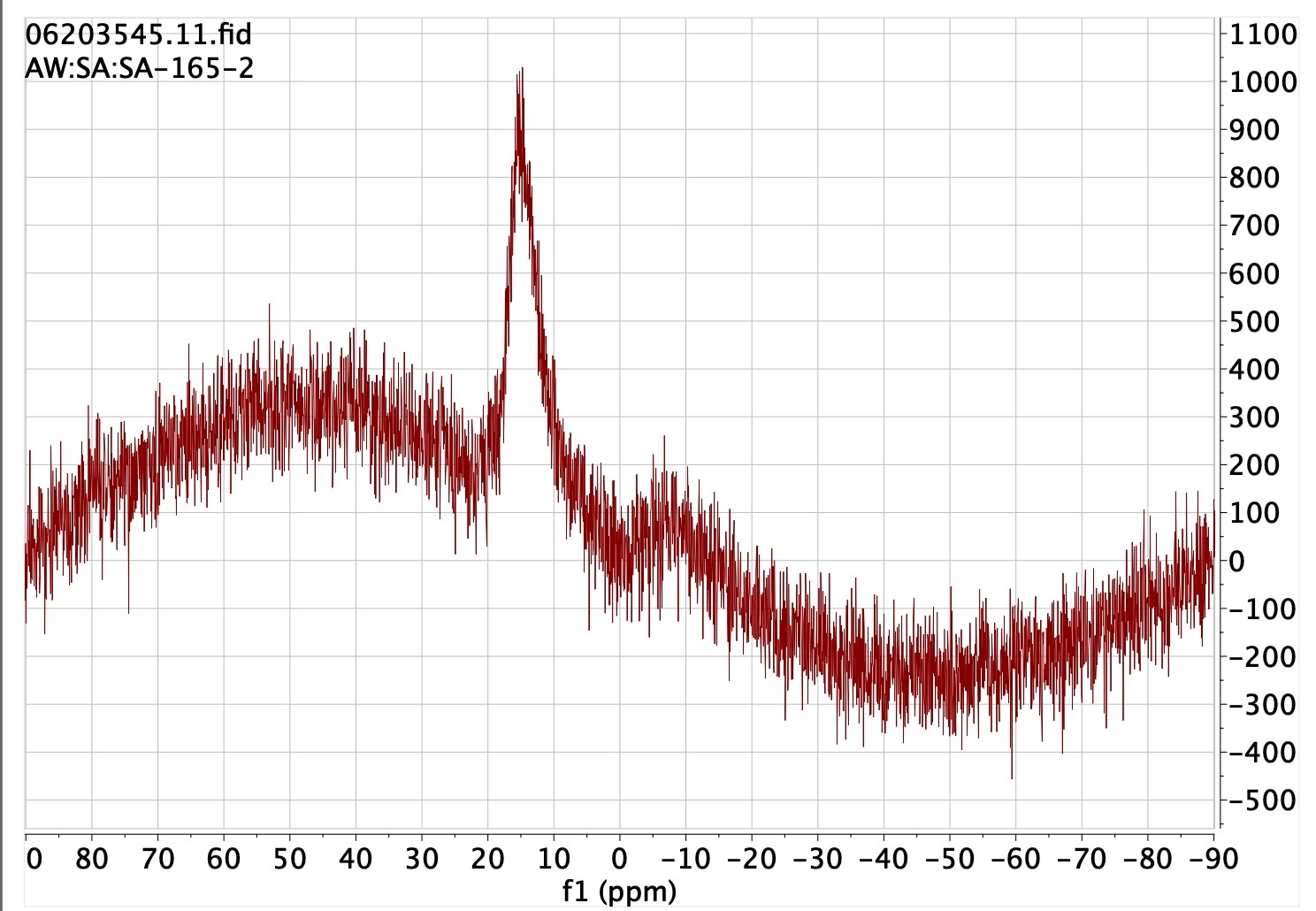
An example is presented of how the metadata associated with the FAIR data items can be used to track down a specific example of this data from any of the 3000 or so data repositories available around the world.
- Download the description of the search here
- You can read an expanded tutorial on the topic here.
- The NMR spectrum Found by this procedure (DOI: 10.14469/hpc/2365) has available a
.mnpub
file which you can download and try producing a spectrum for yourself.
Example 4. How FAIR data could help enable machine learning and artificial intelligence.
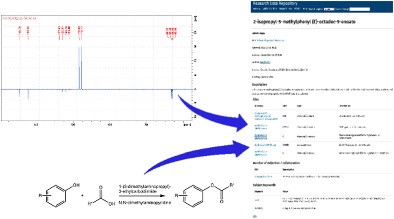
The examples above illustrate not only how an interested human can exploit FAIR data, but also that the entire system has been designed to also allow an unsupervised machine to Find, Access and then Reuse the data for Inter-operable processes such as machine learning. A more complete discussion of these procedures is available.11
Historical postscript to our own FAIR adoption
In 2006 we had decided that the best way to make our research data available was to acquire a data repository. We described our experiences12 and started to learn how to best produce metadata to describe our data. After a decade of using this system, we decided that the next level could only be achieved by designing a repository ourselves that would exploit metadata to a fuller potential.13 We also started to cite research publications by including a data-specific citation alonside the journal citation. Most journal articles now include a data availability section which gives this information, as well as a specific citation to the data in the bibliography. A longer historical perspective of the journey towards FAIR data is also available.14
References
- B. Mons et al, Scientific Data, 2016, DOI: 10.1038/sdata.2016.18
- S. Arkhipenko, M. T. Sabatini, A. S. Batsanov, V. Karaluka, T. D. Sheppard, H. S. Rzepa and A. Whiting, Chem. Sci., 2018, DOI: 10.1039/C7SC03595K, dataDOI: 10.14469/hpc/1620
- Data DOI: 10.14469/hpc/2658
- Data DOI: 10.14469/hpc/2247
- A. Barba, S. Dominguez, C. Cobas, D.P. Martinsen, C. Romain, H. S. Rzepa, F. Seoane, ACS Omega, 2019, 4, 3280-3286. DOI: 10.1021/acsomega.8b03005, dataDOI: 10.14469/hpc/4751
- Data DOI: 10.14469/hpc/2248
- Data DOI: 10.14469/hpc/3177
- Data DOI: 10.14469/hpc/8391
- Data DOI: 10.14469/hpc/1916
- Data DOI: 10.14469/hpc/8396
- H. S. Rzepa and S. Kuhn, Mag. Res. Chem., 2021, DOI: 10.1002/mrc.5186
- J. Downing, P. Murray-Rust, A. P. Tonge, P. Morgan, H. S. Rzepa, F. Cotterill, N. Day and M. J. Harvey, J. Chem. Inf. Mod., 2008, 48, 1571 - 1581. DOI: 10.1021/ci7004737
- M. J. Harvey, A. McLean, H. S. Rzepa, J. Cheminform., 2017, DOI: 10.1186/s13321-017-0190-6, dataDOI: 10.14469/hpc/1088
- H. S. Rzepa, Israel J. Chem., 2021, DOI: 10.1002/ijch.202100034.
Tel: +44 (0)20 7679 2467 Email: tom.sheppard@ucl.ac.uk